Overview
- This visual SLAM benchmark is based on the FusionPortable dataset, which covers a variety of environments in The Hong Kong University of Science and Technology campus by utilizing multiple platforms for data collection. It provides a large range of difficult scenarios for Simultaneous Localization and Mapping (SLAM).
- All these sequences are characterized by structure-less areas and varying illumination conditions to best represent the real-world scenarios and pose great challenges to the SLAM algorithms which were verified in confined lab environments. Accurate centimeter-level ground truth of each sequence is provided for algorithm verification. Sensor data contained in the dataset includes 10Hz LiDAR point clouds, 20Hz stereo frame images, high-rate and asynchronous events from stereo event cameras, 200Hz acceleration and angular velocity readings from an IMU, and 10Hz GPS signals in the outdoor environments.
- Sensors are spatially and temporally calibrated.
- For more information, we can visit the following websits:
Dates
- [08.01]: Training, validation and testing data released
- [09.30]: Result submission deadline
- [10.16]: Challenge results released
- [10.16]: Winner presents
- The deadline for the competition is 24:00 on September 15th, BEIJING time
Notice
A team can only register one account. Quota can be obtained by joining the WeChat group.
In order to prevent the problem of a team registering multiple accounts, this competition requires all members of the participating team to join the WeChat group. If the QR code is invalid, we will update it in time. And the old account cannot be used, you need to re-register a new account.
If you do not have WeChat, please send your application to tju.drone.vision@gmail.com. The application information should include account name, real name, institution, country, email address and the name and institution of team members.
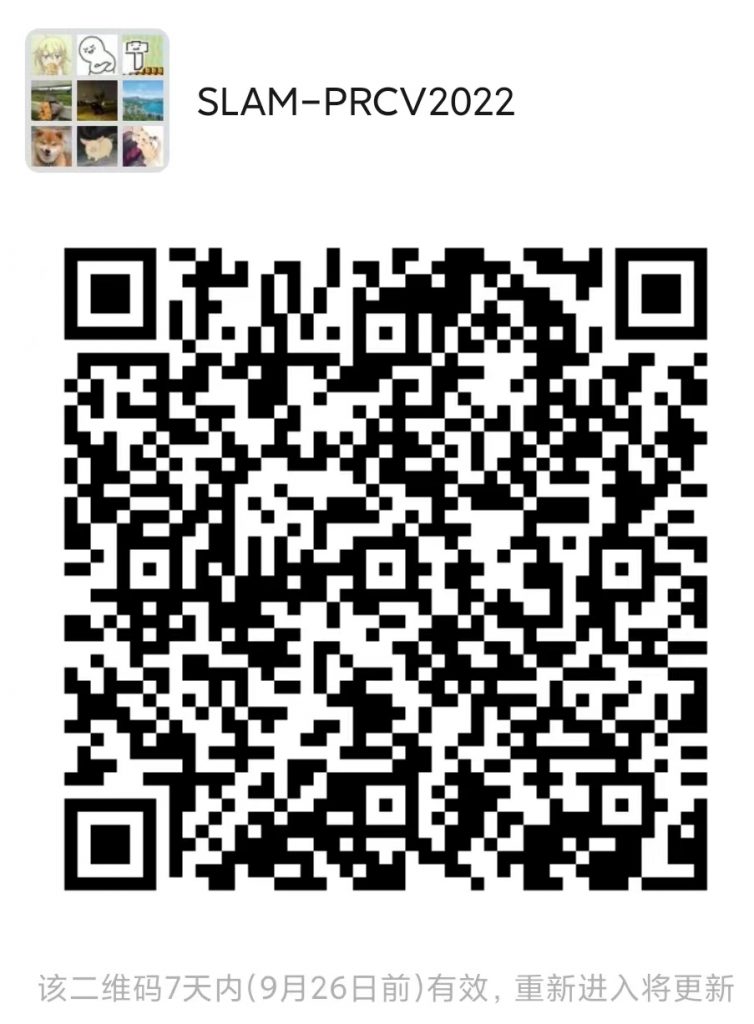
Hardware
- The sensors are mounted rigidly on an aluminium platform for handheld operation. An FPGA is utilized to generate an external signal trigger to synchronize clocks of all sensors. We install the sensor rig on various platforms to simulate distinguishable motions of different equipments, including a handheld device with a gimbal stabilizer, a quadruped robot, and an autonomous vehicle.
Sensor | Characteristics |
3D LiDAR (not provided) | Ouster OS1-128, 128 channels, 120m range |
Frame Camera * 2 | FILR BFS-U3-31S4C, resolution: 1024 × 768 |
Event Camera * 2 | DAVIS346, resolution: 346 × 240,2 built-in imu |
IMU (body_imu) | STIM300 |
GPS | ZED-F9P RTK-GPS |
Ground Truth | Leica BLK 360 |
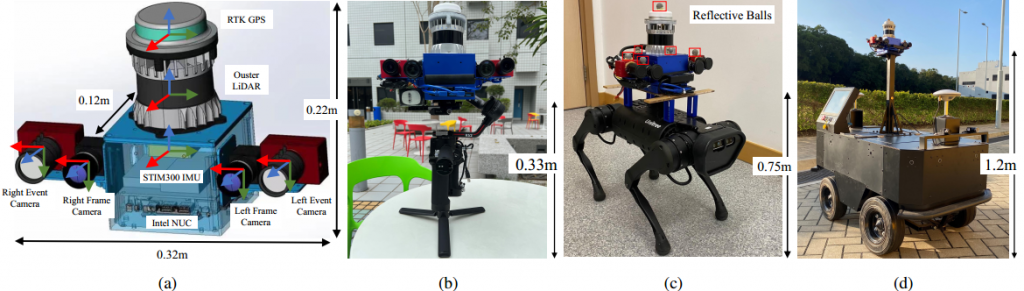
- Calibration: The calibration file in yaml format can be downloaded here. We provide intrinsics & extrinsics of cameras as well as noise parameters of the IMU and also the raw calibration data. Intriniscs are calibrated using the MATLAB tool, and the extrinsics are calibrated using the Kalibr. Taking the frame_cam00.yaml as an example, parameters are provided in the form as follows:
image_width: 1024
image_height: 768
camera_name: stereo_left_flir_bfsu3
camera_matrix: !!opencv-matrix
rows: 3
cols: 3
dt: f
data: [ 6.05128601e+02, 0., 5.21453430e+02,
0., 6.04974060e+02, 3.94878479e+02,
0., 0., 1. ]
...
# extrinsics from the sensor (reference) to bodyimu (target)
quaternion_sensor_bodyimu: !!opencv-matrix
rows: 1
cols: 4
dt: f
data: [0.501677, 0.491365, -0.508060, 0.498754] # (qw, qx, qy, qz)
translation_sensor_bodyimu: !!opencv-matrix
rows: 1
cols: 3
dt: f
data: [0.066447, -0.019381, -0.077907]
timeshift_sensor_bodyimu: 0.03497752745342453
Rotational and translational calibration parameters from the camera (reference frame) to the IMU (target frame) are presented in the form of the Hamilton quaternion ([qw, qx, qy, qz]) and the translation vector ([tx, ty, tz]). The timeshift is obtained by the Kalibr.